In collaboration with Griffith University and The University of Otago, today we have published a major new study in PLOS Climate today on the data quality of Scope 3 and the ability to predict emissions using machine learning techniques.
Scope 3 emissions are indirect emissions that occur in a company's value chain but are not owned or controlled by the company. These emissions result from 15 different categories like business travel, the purchased goods and services, processing and use of sold products or the disposal of waste generated by the company's operations.
Scope 3 emissions often dominate a company's total carbon footprint, particularly for financial institutions, energy, utilities, mining and materials companies. These emissions are difficult to quantify but critical in understanding how companies are financially exposed to carbon pricing and their decarbonisation pathways.
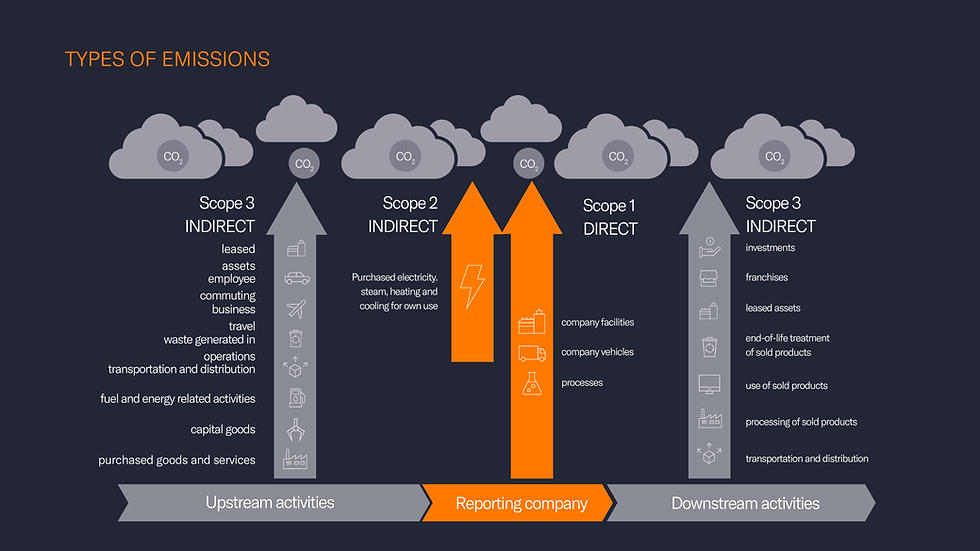
Over the last few years we have invested into a research collaboration with leading climate financial researchers to better understand and predict Scope 3 emissions as they are an essential investment tool for climate-aware investors to understand their climate transition risk. This new paper analysed reported Scope 3 emissions for 1,972 companies up until 2019.
Key Research Takeaways
The most carbon intensive categories were significantly under-reported by most companies. For example by 2019, 86% of firms disclosed travel emissions but only 24% disclosed emissions from ‘Use of Sold Products’. Travel emissions account for just 0.3% of Scope 3 emissions - while we found Use of Sold Products accounted for 63%, vastly more important than any other category.
We found most companies are significantly under-reporting their Scope 3 emissions by up to 44%. In 2019 we estimate that ~7billion tonnes of Scope 3 emissions are under-reported by companies.
We found machine learning models that predict Scope 3 aggregate only marginally improve (~6%) the prediction accuracy of traditional methods. However machine learning significantly improved (up to 25%) when predicting for each of the 15 categories. Improved reported is important to increase the accuracy of machine learning models for Scope 3.
Although significant uncertainty remains, our novel machine learning approach to estimating Scope 3 emissions is valuable to understand whether a company has 'material' financial exposure to a lower carbon world where carbon is legislated and priced.
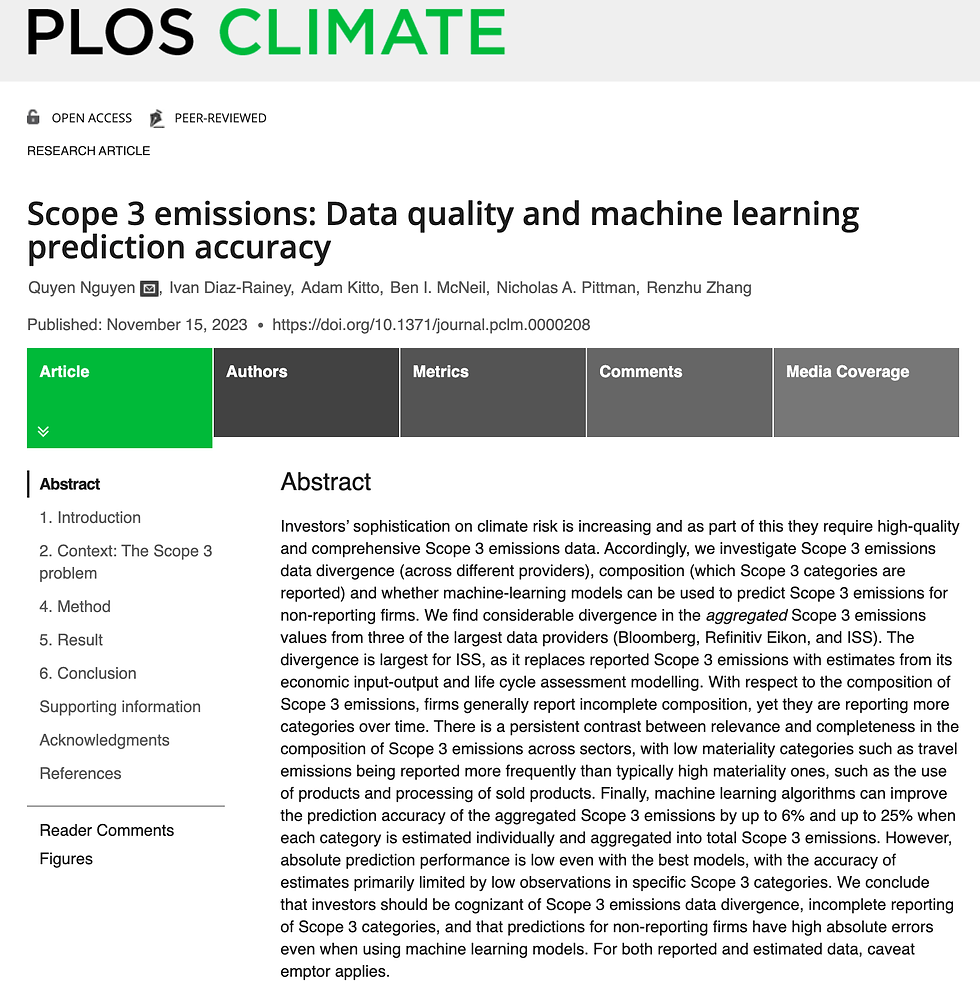
Kommentit